- Agile
- Artificial Intelligence Engineering
- CERT/CC Vulnerabilities
- Cloud Computing
- CSIRT Development
- Cyber Workforce Development
- Cyber-Physical Systems
- Cybersecurity Engineering
- DevSecOps
- Edge Computing
- Enterprise Risk and Resilience Management
- Insider Threat
- Quantum Computing
- Reverse Engineering for Malware Analysis
- Secure Development
- Situational Awareness
- Software Architecture
- Software Engineering Research and Development
- Technical Debt
The Great Fuzzy Hashing Debate
This post details a debate among two researchers over whether there is utility in applying fuzzy hashes to instruction bytes.
• By Edward J. Schwartz
In Reverse Engineering for Malware Analysis
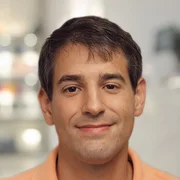
Comparing the Performance of Hashing Techniques for Similar Function Detection
This blog post explores the challenges of code comparison and presents a solution to the problem.
• By Edward J. Schwartz
In Reverse Engineering for Malware Analysis
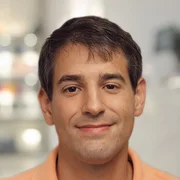
The Latest Work from the SEI: an OpenAI Collaboration, Generative AI, and Zero Trust
This post highlights the latest work from the SEI in the areas of generative AI, zero trust, large language models, and quantum computing.
• By Douglas Schmidt (Vanderbilt University)
In Software Engineering Research and Development
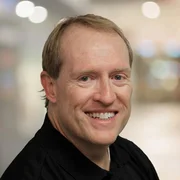
Applying Large Language Models to DoD Software Acquisition: An Initial Experiment

This SEI Blog post illustrates examples of using LLMs for software acquisition in the context of a document summarization experiment and codifies the lessons learned from this experiment and related …
• By Douglas Schmidt (Vanderbilt University), John E. Robert
In Artificial Intelligence Engineering
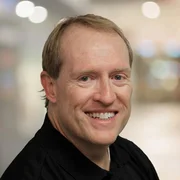
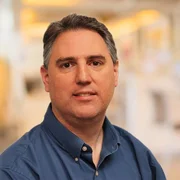
5 Recommendations to Help Your Organization Manage Technical Debt
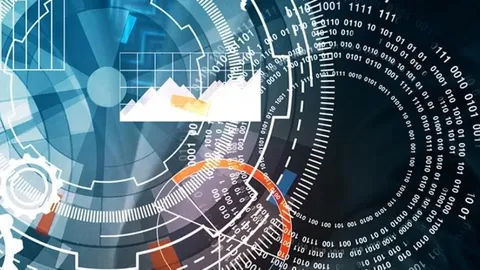
This SEI Blog post summarizes recommendations arising from an SEI study that apply to the Department of Defense and other development organizations seeking to analyze, manage, and reduce technical debt.
• By Ipek Ozkaya, Brigid O'Hearn
In Technical Debt
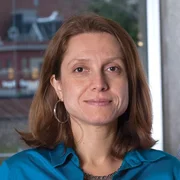
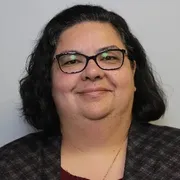
API Security through Contract-Driven Programming
This blog post explores contract programming and specifically how that applies to the building, maintenance, and security of APIs.
• By Alex Vesey
In Cybersecurity Engineering
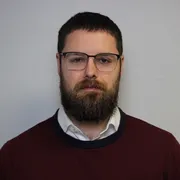
Example Case: Using DevSecOps to Redefine Minimum Viable Product
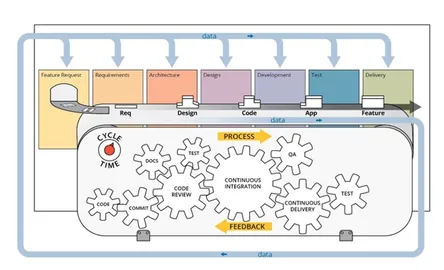
This SEI blog post, authored by SEI interns, describes their work on a microservices-based software application, an accompanying DevSecOps pipeline, and an expansion of the concept of minimum viable product …
• By Joe Yankel
In DevSecOps
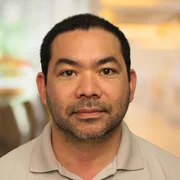
10 Lessons in Security Operations and Incident Management
This post outlines 10 lessons learned from more than three decades of building incident response and security teams throughout the globe.
• By Robin Ruefle
In Insider Threat
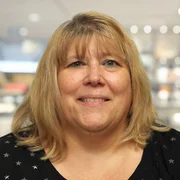
CERT Releases 2 Tools to Assess Insider Risk
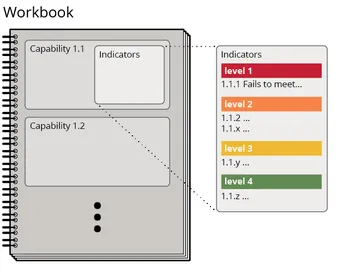
The average insider risk incident costs organizations more than $600,000. To help organizations assess their insider risk programs, the SEI CERT Division has released two tools available for download.
• By Roger Black
In Insider Threat
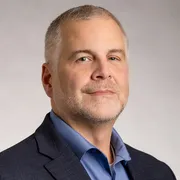
OpenAI Collaboration Yields 14 Recommendations for Evaluating LLMs for Cybersecurity
This SEI Blog post summarizes 14 recommendations to help assessors accurately evaluate LLM cybersecurity capabilities.
• By Jeff Gennari, Shing-hon Lau, Samuel J. Perl
In Artificial Intelligence Engineering
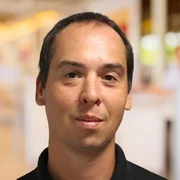
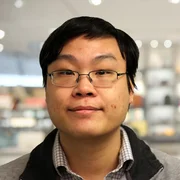
Explore Topics
- Agile
- Artificial Intelligence Engineering
- CERT/CC Vulnerabilities
- Cloud Computing
- CSIRT Development
- Cyber Workforce Development
- Cyber-Physical Systems
- Cybersecurity Engineering
- DevSecOps
- Edge Computing
- Enterprise Risk and Resilience Management
- Insider Threat
- Quantum Computing
- Reverse Engineering for Malware Analysis
- Secure Development
- Situational Awareness
- Software Architecture
- Software Engineering Research and Development
- Technical Debt
Get updates on our latest work.
Sign up to have the latest post sent to your inbox weekly.
Subscribe Get our RSS feedGet updates on our latest work.
Each week, our researchers write about the latest in software engineering, cybersecurity and artificial intelligence. Sign up to get the latest post sent to your inbox the day it's published.
Subscribe Get our RSS feed