SEI Researchers Help Design xView2 Machine Learning Competition
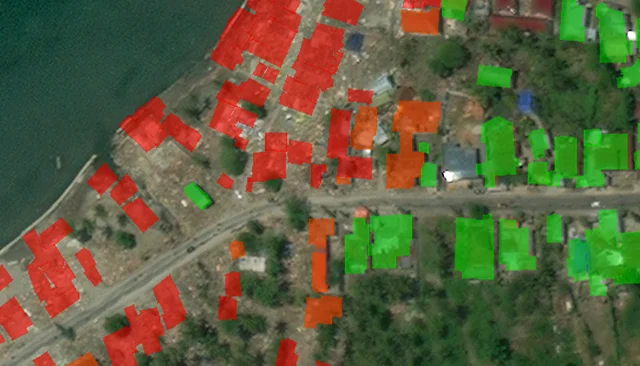
• Article
September 24, 2019—Researchers from the SEI’s Emerging Technology Center (ETC) recently completed work underpinning a just-opened machine learning (ML) competition. The xView2 Challenge, from the Department of Defense’s Defense Innovation Unit (DIU), tests computer vision algorithms’ ability to perform disaster damage assessment based on satellite imagery.
The xView2 Challenge asks ML experts to use computer vision to scan satellite images of buildings before and after disasters, including wildfire, landslides, dam collapses, volcanic eruptions, earthquakes, tsunamis, wind, and floods. The algorithms must automatically find buildings and then classify their damage. DIU hopes to foster solutions that will speed on-the-ground recovery efforts following natural or human-caused disasters.
Ritwik Gupta, an ML research scientist in the SEI’s ETC, and other members of the SEI garnered a fifth-place finish in the xView 1 challenge, which focused on improving computer vision’s fine-grained, dense object recognition in satellite images. Gupta kept in touch with the DIU after xView 1, and the sponsors asked him and his colleagues to help design the next challenge.
This January, Gupta and Ricky Hosfelt, a software engineer in the ETC, started curating imagery of pre- and post-disaster buildings from Maxar/DigitalGlobe’s Open Data Program, a provider of openly licensed imagery for disaster response. The researchers, DIU, and collaborators from the humanitarian assistance and disaster recovery (HADR) community created the Joint Damage Scale to enable consistent labeling of damage across different types of disasters, structures, and geographies.
The next step was to label the buildings in the post-disaster images with a degree of damage, using the Joint Damage Scale, and the cause: wildfire, flood, wind, earthquake or tsunami, or volcanic eruption. Gupta and Hosfelt guided that labeling effort, which was crowdsourced through CrowdAI, and quality checked the labels with HADR partners and experts in satellite imagery and remote sensing. The final result, called xBD, is one of the largest satellite imagery datasets for building damage assessment, including more than 550,000 labeled buildings over 7,335 square miles.
The xBD dataset is expected to be a breakthrough source of training data for the nascent field of applied ML and artificial intelligence (AI) in HADR. For the xView2 Challenge, the xBD dataset constitutes the training data for the competitors’ ML algorithms and the ground truth against which their automatically generated damage assessments will be compared.
Gupta, Hosfelt, and SEI interns Danny Tunitis and Sandra Sajeev also created and tested dozens of damage-assessment ML algorithms that partially perform the xView2 Challenge tasks. Some of those reference algorithms will be made available to xView2 competitors as an optional starting point.
The DIU recently opened the xView2 Challenge by making the xBD dataset available to download. Competitors will modify the starting ML algorithms or create their own to classify building damage in the curated satellite imagery. Their results will be compared to the ground truth from the xBD dataset, according to metrics designed by Gupta and Hosfelt. Teams with the most similar results will win prize money from a pool of $150,000. Winners will be given an opportunity to present at the AI + HADR Workshop, which is chaired by Gupta and will be held during December’s NeurIPS 2019 Conference in Vancouver, B.C.
Hosfelt is excited to help push the boundaries of technology in HADR. “We can potentially provide first responders with enhanced information to save victims of natural disasters and help keep responders safe.”
Gupta hopes to contribute to other practical competitions in the future. “We want these challenges to make a difference to agencies and their partners in their day-to-day operations,” he said. “We want to make sure these competitions are academically challenging but operationally relevant.”