Foundational Research Behind Text Analytics for Insider Threat: Part 2 of 3
PUBLISHED IN
Insider ThreatIn this blog series, I review topics related to deploying a text analytics capability for insider threat mitigation. In this segment, I continue the conversation by disambiguating terminology related to text analysis, summarizing methodological approaches for developing text analytics tools, and justifying how this capability can supplement an existing capability to monitor insider threat risk. In my next post, Acquiring or Deploying a Text Analytics Solution, I will discuss how organizations can think through the process of procuring or developing a custom in-house text analytics solution.
Sentiment Analysis Versus Affect Analysis Versus Text Analysis?!
You may be scratching your head after reading the first post in this series about text analytics. What is the relationship between text analytics, affect analysis (which I'm guessing is a new term), and sentiment analysis? Below is a brief list of the (seemingly ambiguous) terminology and some definitions. Each of these approaches can analyze text from a cyber collection capability.
Broad Terminology
- Text analysis is the process of analyzing written textual data for a specific task such as affect, sentiment, linguistic, or topic analysis (sometimes referred to as content analysis).
- Content analysis is the process of quantitatively analyzing the content of language.
Applications
- Sentiment analysis is the task of classifying text into polarity (positive or negative) states.
- Linguistic analysis is the study of grammar, syntax, and semantics for the purpose of modeling language.
- Affect analysis is the task related to identifying and measuring emotion, sentiment, personality, mood, and interpersonal stances. Affect constructs vary in intensity, duration, behavioral expression, and impact.
Figure 1 shows a working model of the relationships between these terms. You can see that both the social and information sciences have contributed to the development of these interdisciplinary areas.
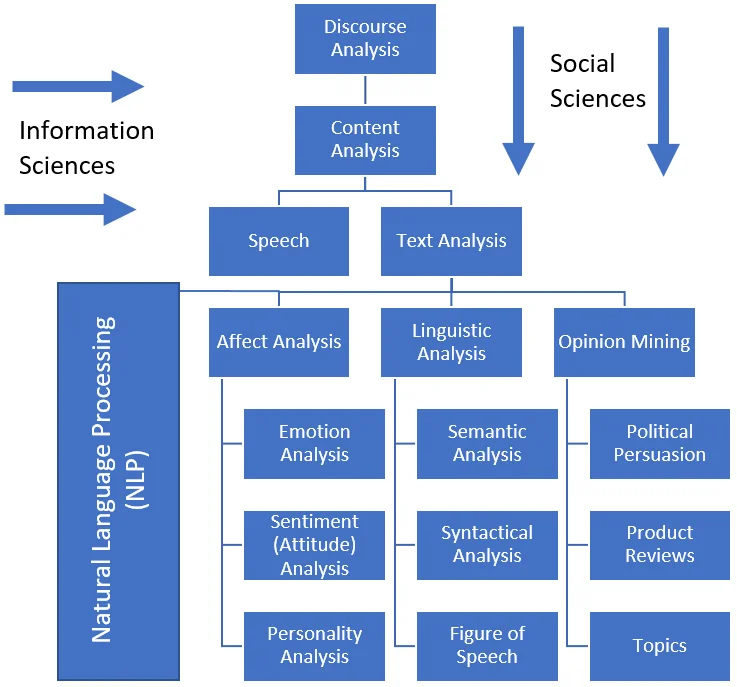
Figure 1 - Taxonomical Representation of the Text Analysis field
Insider threat researchers, practitioners, and developers primarily use the terms text analytics and text analysis to refer to the broad category of using textual data (such as employer-owned email or instant messages) to measure or identify a specific affect construct (e.g., user sentiment or emotion).
Features of affect that could be pertinent to insider risk assessment include:
Additionally, see Predictive Modeling for Insider Threat Mitigation for another list of psycho-social observations related to insider threat risk.
Methodological Approaches
Let's do a brief deep-dive into the theoretical background of approaches related to text analytics. Schwartz and Ungar frame the methods and analytical techniques that underpin text analytics as a continuum of a hypothesis-driven to a data-driven approach (see Figure 2). Hypothesis-driven approaches developed from manual coding of terms to word associations based upon prior theories or postulations.
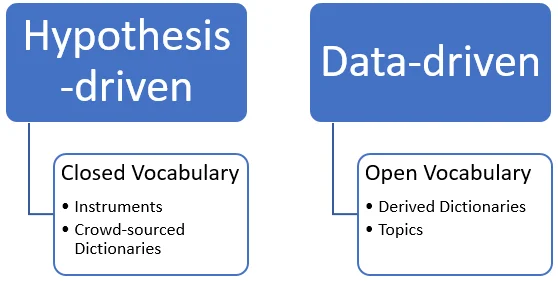
Figure 2 - Text Analysis Approaches. Adapted from: Schwartz, H. A., & Ungar, L. H. (2015).
An example of a hypothesis-driven instrument is the software application LIWC (Linguistic Inquiry and Word Count, pronounced "luke"). LIWC is a text analytics tool known for its inter-rater reliability, multi-language support, and multi-application use. (Google Scholar lists over 5,000 citations for two of the primary LIWC references.) Many studies across a wide range of domains and languages have utilized LIWC as the primary or sole instrument to measure affect constructs. The breadth of usage provides supporting evidence of the validity and dependability of the instrument's precision and accuracy.
The state of the art in text analytics as a whole has seen data-driven approaches flourish. Advanced natural language processing (NLP) and computer automation techniques facilitate the ability to automate textual analysis and derive insight from data sets. These data-driven approaches rely on machine learning techniques, such as the supervised development of derived dictionaries or unsupervised topic models to extract categories.
However, for now and the foreseeable future, text analytics use cases for insider threat are founded on an instrument-based approach that uses a derived dictionary built and tuned from multiple studies. Instrument-based approaches are typically easier to implement and interpret, due to an existing body of research and the number of automated off-the-shelf solutions available to deploy. Future work in insider threat use cases can build upon data-driven approaches (like topic models) to extract measurements and insight.
Why Incorporate Affect Analysis into Insider Risk Monitoring?
The simple answer: it provides another data point.
Measuring insider risk is about collecting and interpreting data to assist decision makers in characterizing the risk environment. The goal is to improve prediction models and mitigation practices. Text analytics provides a rich data point for understanding the context of events related to how users interact with organization-owned assets, allowing for more informed evaluations. Supporting literature, experimental studies, and real-world applications show their effectiveness. We do not claim that text analytics provides a complete or comprehensive account, only that it provides additional intelligence for analysts or your analytics tool (such as a User Behavior Analytics (UBA) or User Behavior and Entity Analytics (UBEA) tool) to digest and analyze. Ultimately, deciding whether to adopt a text analytics capability is a cost-benefit decision that each organization must consider and decide for itself.
Stay tuned for the next blog segment, Acquiring or Deploying a Text Analytics Solution, to learn more about how organizations can think through the process of procuring or developing a customized, in-house text analytics capability.
Please send questions, comments, or feedback to insider-threat-feedback@cert.org.
More By The Author
More In Insider Threat
PUBLISHED IN
Insider ThreatGet updates on our latest work.
Sign up to have the latest post sent to your inbox weekly.
Subscribe Get our RSS feedMore In Insider Threat
Get updates on our latest work.
Each week, our researchers write about the latest in software engineering, cybersecurity and artificial intelligence. Sign up to get the latest post sent to your inbox the day it's published.
Subscribe Get our RSS feed