Creating Transformative and Trustworthy AI Systems Requires a Community Effort
As the SEI leads the community effort toward human-centered, robust, secure, and scalable AI, we are learning what is needed to move toward transformative and trustworthy AI systems. In this post, we describe how professionalizing the practice of AI engineering and developing the AI engineering discipline can increase the dependability and availability of AI systems. We also share what’s needed in the AI engineering community and how to get involved.
Voices calling for an AI engineering discipline are growing. Government entities such as the Defense Innovation Unit (DIU) are launching initiatives like the Responsible AI Guidelines to embed trust and social responsibility into DoD AI innovation activities. On a related front, research entities such as the IEEE Computer Society (CS) are launching special issue journals like AI Engineering to share practical experiences and research results for developing AI-intensive systems. Similarly, private sector entities including IBM and Coursera are partnering to release educational programming to train workforce members to build transformative and trustworthy AI systems. In addition to these institutional efforts, researchers such as Hannah Kerner, James Llinas, and Andrew Moore are championing the need for an applied discipline of AI engineering.
In partnership with the Office of the Director of National Intelligence (ODNI), we at the Carnegie Mellon University (CMU) Software Engineering Institute (SEI) are leading a national initiative to advance the discipline of AI engineering to increase utility and dependability of AI systems. We have hosted workshops and a symposium, published white papers and software artifacts, and shared resources on how to produce human-centered, robust and secure, and scalable AI systems. In the months ahead, we will continue to grow the AI engineering community by hosting discussions and fostering collaborations. With this work more than a year underway, we would like to share some insights we’ve gained and invite ideas and feedback in this blog post.
AI Systems Need to Shift from Brittle to Dependable
Organizations of all sizes and across all sectors are investing in AI technologies at an unprecedented rate to transform business and mission outcomes and to unlock competitive advantages. These AI investments are increasingly being implemented in high-stakes and high-availability scenarios, requiring sophisticated reliability engineering for operational assurance and responsible usage. Unfortunately, the return on AI investments is remarkably risky – Gartner estimates that nearly 85 percent of AI projects will fail in 2022. AI incident trackers, such as the AI Incident Database (AIID), are cataloging associated harms from failed AI endeavors (such as the self-driving Uber crash) and capturing examples of the real, sometimes irreversible, damage caused by brittle AI systems.
Incidents in the AIID, along with the examples of AI deployed in high-stakes and high-availability scenarios, call for shifting the mindset of AI system development from an ad hoc craft to a dependable engineering practice that is optimized to maximize value and minimize risk associated with the engineering construction. Traditional engineering disciplines have turned to practice professionalization as an enabler for optimizing this balance at a societal level.
Professionalizing the Practice is One Way Forward
Practice professionalization serves to standardize expectations for the performance of services and provides increased protections and channels for resolving issues. Consider the trust we place in our doctors, our lawyers, and even the engineers who design and construct our homes. We rely on their expertise to ensure that the products and services we receive are dependable and useful. It has become increasingly clear that society seeks to rely upon AI systems embedded in everyday infrastructure, including in high-stakes and high-availability applications, such as recommender systems in judicial sentencing, object detection systems in satellite surveillance, and optimization systems in financial forecasts.
As organizations integrate AI technology into these complex systems, rigorous engineering is required to balance system design tradeoffs and to avoid unintended consequences. Professional engineering practices (such as civil engineering) cultivate and uphold these rigorous standards (such as structural safety requirements) to facilitate quality engineering. Professional practice resources, such as certifications, accreditation systems, codes of practice, and professional development, offer vehicles to mature the collective state of the practice. For AI engineering, professional practice resources will provide practitioners tools to integrate AI technology into complex and dynamic systems (such as test and evaluation criteria for continuous ML monitoring).
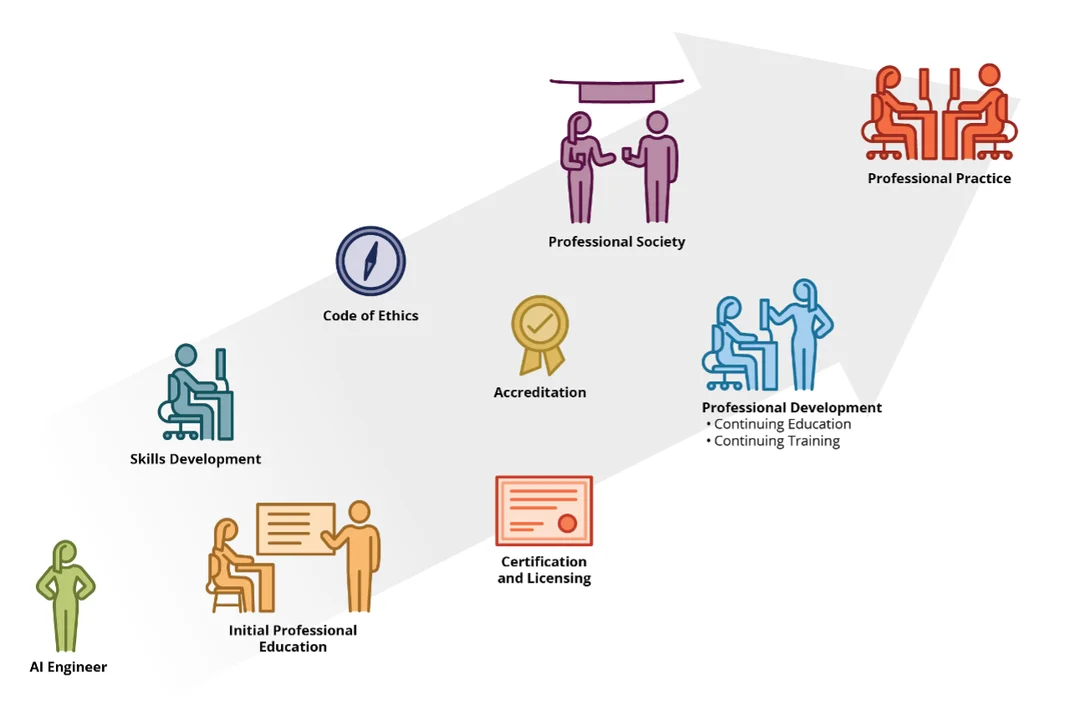
Formally embarking on practice professionalization is a long-term, community-based effort. In the meantime, works such as the DIU Responsible AI Guidelines and NIST AI Risk Management Framework provide practitioner resources to increase practice dependability. The bottom line is that we need to increase the rigor of AI engineering standards. Not every project will have high-stakes and high-availabiity requirements, but the mechanisms to adapt dependability requirements are needed, and this work begins with formalizing the engineering discipline.
Scale AI Delivery with an Engineering Discipline
We can scale the delivery of AI across contexts by curating engineering resources, such as frameworks, tools, and processes. Much like civil engineering relies upon engineering discipline tools (such as structural scaffolding for building commercial buildings) and frameworks (such as structural analysis for selecting safe and sustainable structural load support), the discipline of AI engineering will provide reference resources to develop and deliver trustworthy and impactful AI systems.
Engineering disciplines are a particular subset of disciplines focused on “creating cost-effective solutions to practical problems by applying scientific knowledge building things in the service of [hu]mankind,” according to a founder of the software engineering discipline, Mary Shaw. Engineers transform society by taking what’s technologically feasible from basic and applied research to both solve routine problems and create innovative solutions. Engineers do more though than simply creating functional real-world designs – engineers work purposefully to create durable and customer-centric systems. An AI engineering discipline allows practitioners a common framework for solving problems and creating solutions, enabling practitioners with more structured tools like MLOps paradigms for innovation.
These engineering discipline building blocks, such as the body of knowledge, reference models and reference architectures, decision frameworks and design patterns, and performance standards, serve as common resources for the AI engineering practice and help scale systems development by allowing for component reuse and interoperability.
Currently, the integration of AI technologies, such as machine learning, into software applications relies heavily upon bespoke systems and product-specific procedures, as adjacent disciplines of software engineering and systems engineering handle related but distinct concerns. These adjacent engineering disciplines need a standardized reference for interfacing with AI engineering. This reference will ensure that these adjacent disciplines make accurate assumptions and requirements for interacting with AI engineering practices and avoid risks associated with component mismatches. Here, the insight is that to take advantage of AI engineering building blocks, we need formalize the process of turning shared tradecraft stories into actionable lessons learned – whether in the form of repeatable practices or avoidable mistakes.
As the AI engineering discipline develops, here are a few challenge questions to consider:
- How might we rapidly benchmark AI systems across operational-readiness levels?
- How might we cultivate an AI engineering body of knowledge?
- How might we minimize the buildup of technical debt in AI systems?
- How might we develop interoperable AI systems?
- How might we quantify and manage AI system risk?
- How might we quantify and manage the sustainability of AI systems?
Increasing Expectations for AI Systems Requires a Community
Growing and professionalizing the discipline of AI engineering requires a professional community in which practitioners can self-identify as AI engineers. Community membership needs representation across producers, consumers, and researchers of AI:
- AI producers, such as digital transformation consultants, machine learning engineers, and integrators, lead the lifecycle of AI system development, the core AI engineering activities.
- AI producers translate the problem into a use case and then transform the raw materials (data, standard libraries, infrastructure) into a final system that meets AI consumer needs.
- AI consumers provide the use case to AI producers and monitor the AI producer performance, measuring what “success” looks like.
- And AI researchers discover “what’s possible” – spotlighting potential opportunities for new ideas and new engineering solutions as well as novel challenges posed by AI in real-world contexts.
Unfortunately, current AI engineering discourse is generally siloed into role-specific mediums, such as producer industry conferences, consumer policy forums, and researcher academic conferences. This siloing results in conversations that are heavily focused on tactical challenges, such as designing scalable, resilient, and interoperable architectures, or optimizing performance and accountability metrics related to model precision and explainability. These challenges are foundational to deploying mission-ready capabilities and are necessary for achieving technological viability. To increase the strategic impact value of AI systems however, AI engineers need cross-functional dialogue and resources related to customer-centric innovation for transformative and trustworthy AI, and that requires combining AI producers, AI consumers, and AI researchers into a “big tent” AI engineering community.
The Road Ahead for AI Engineering
Looking to the road ahead for AI engineering, we see some short-term and long-term activities and milestones. In the short-term, we need to cultivate a diverse community of people engaged in all aspects AI engineering work. This community of interest should begin the co-development of the AI engineering body of knowledge and code of ethics. In the short term, this community should also identify archetype roles of the AI engineering process, considering what competencies and responsibilities individuals in these roles should have. In the long-term, as the community of interest matures, working groups should be tasked to pursue accreditation standards, certifications, and formal role standardization. Consideration should also be given to the idea of practice professionalization, and what form that may take for AI engineering.
With that, a few final thoughts to wrap up this post:
- We would love to hear your feedback and thoughts on this topic. You can reach our team at ai-eng@sei.cmu.edu or send us a message here.
- With the great turnout and feedback from our AAAI Spring Symposium, we are seeking to launch a monthly speaker series this summer, leading up to another multi-day event in the fall. If you are interested in speaking or participating, let us know.
- Want to meet with us? Sign up to attend office hours and speak with Carrie Gardner and Rachel Dzombak.
Additional Resources
- On May 18, Matthew Butkovic will talk with Michael Mattarock, a senior engineer in program development in the SEI’s AI Division, about the SEI’s efforts to apply AI techniques to address national security mission needs while leading a national initiative to build a new discipline of AI Engineering. For more information or to register https://www.linkedin.com/video/event/urn:li:ugcPost:6924756924609556480/
- Read the blog post from Rachel Dzombak and Jay Palat, 5 Ways to Start Growing an AI-Ready Workforce
- Read Rachel Brower-Sinning’s post, Improving Automated Retraining of Machine-Learning Models.
- Read Brett Tucker’s white paper, A Risk Management Perspective for AI Engineering.
More By The Author
Insider Threats in Entertainment (Part 8 of 9: Insider Threats Across Industry Sectors)
• By Mark Dandrea, Carrie Gardner
More In Artificial Intelligence Engineering
PUBLISHED IN
Get updates on our latest work.
Sign up to have the latest post sent to your inbox weekly.
Subscribe Get our RSS feedMore In Artificial Intelligence Engineering
Get updates on our latest work.
Each week, our researchers write about the latest in software engineering, cybersecurity and artificial intelligence. Sign up to get the latest post sent to your inbox the day it's published.
Subscribe Get our RSS feed