Five Models of Technology Transition to Bridge the Gap Between Digital Natives and Digital Immigrants
As the defense workforce attracts younger staff members, this digital native generation is having an effect. "To accommodate millennial IT workers, so-called 'digital natives,'" wrote Phil Goldstein in a May 2016 FedTech article, "the service branches of the Department of Defense need to square cybersecurity with the attitudes and behaviors of younger employees, according to senior defense IT officials." Digital natives approach technology differently than digital immigrants, which includes those born before the widespread use of technology. In this blog post, I explore five classic transition models to determine what, if any, considerations we need to account for in today's environment that are different from when they were first published, many of them before the digital natives phenomenon was identified.
The five models are related to technology transition and adoption, and they answer the following questions:
- What kind of technology is it?
- How big is the adoption being contemplated?
- Who will be adopting the new technology?
- What must change agents or technologists do to improve the chance of the technology's success?
- How do we help people get from their current environment to one that leverages the new technology?
Each of these questions is supported by one or more 20th century transition models. Some are still useful as is; others may need to be adapted to the current environment. The observations about digital natives and digital immigrants come from my personal observations over the last 15 years in working with both populations, primarily transitioning practice-based technologies, such as Agile methods.
What Kind of Technology Is It?
There are lots of ways to categorize technologies. Two have been particularly useful for us at the SEI.
The first involves categorizing technologies as hardware, software, or practice-based. The first two categories are obvious. The third concerns changes in the engineering or management practices that are large enough to pose many of the same challenges, as hardware and software (e.g., secure coding) technology transition.
A second useful way to categorize technologies is the Winds of Creative Destruction model by William Abernathy and Kim Clark. This model differentiates technologies in terms of their economic impact:
- Architectural technologies drastically change how something is produced and how it is discussed or promoted in the marketplace.
- Revolutionary technologies drastically change how something is produced, but don't radically change how it's discussed.
- Niche technologies don't radically change how something is produced, but do drastically change how it's discussed.
- Evolutionary technologies don't radically change either how something is produced or how it's discussed.
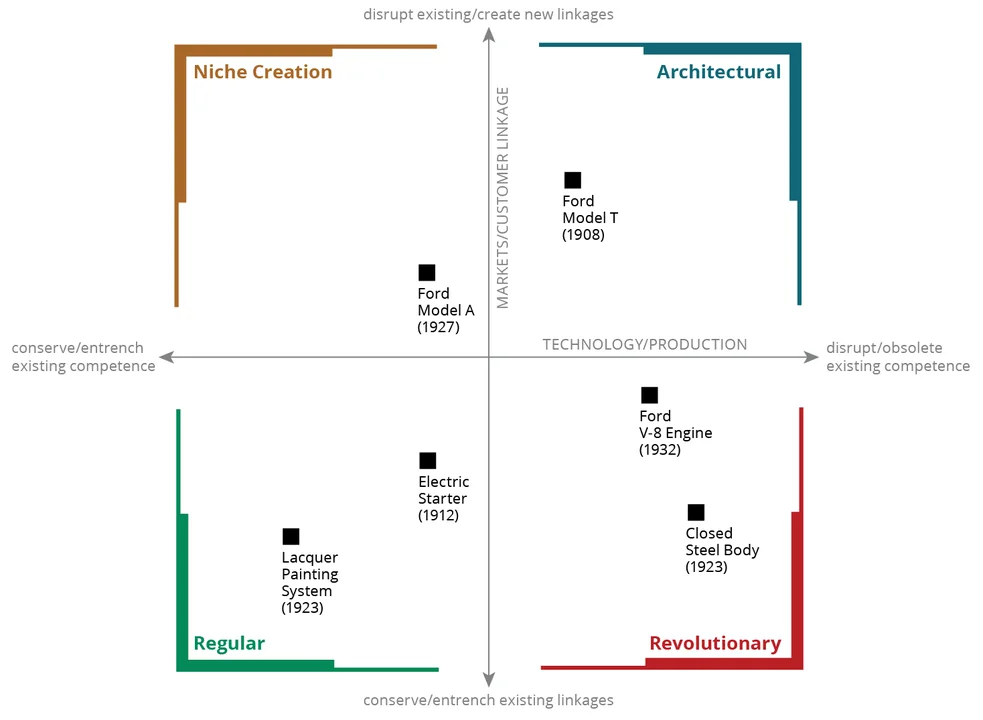
Adapted from Innovation: Mapping the winds of creative destruction by William J. Abernathy and Kim Clark.
Practice-based technologies tend to be evolutionary or revolutionary. The title comes from the observation that architectural technologies destroy both marketing and production infrastructure and therefore require a much longer adoption cycle unless there is an urgent, dramatic need for the architectural technology in question.
When thinking about these four types of technologies in the context of digital immigrants and digital natives, it is important to remember that each cohort has differing perceptions of what constitutes a change in the means of production. The other difference lies in communication and marketing channels. In particular, the use of various social media channels is likely to be a baseline communication and/or marketing approach for digital natives, whereas digital immigrants don't typically engage as much in social media.
How big is the adoption being contemplated?
Architectural technologies often require large changes to implement them and create significant effects on the adopting organization. Paul Adler, a researcher in technology adoption, has characterized the size of technology change to help organizations understand how long it may take to achieve the change. Adler uses aspects of the organization that need to change to accommodate the new technology as the way for talking about "bigness."
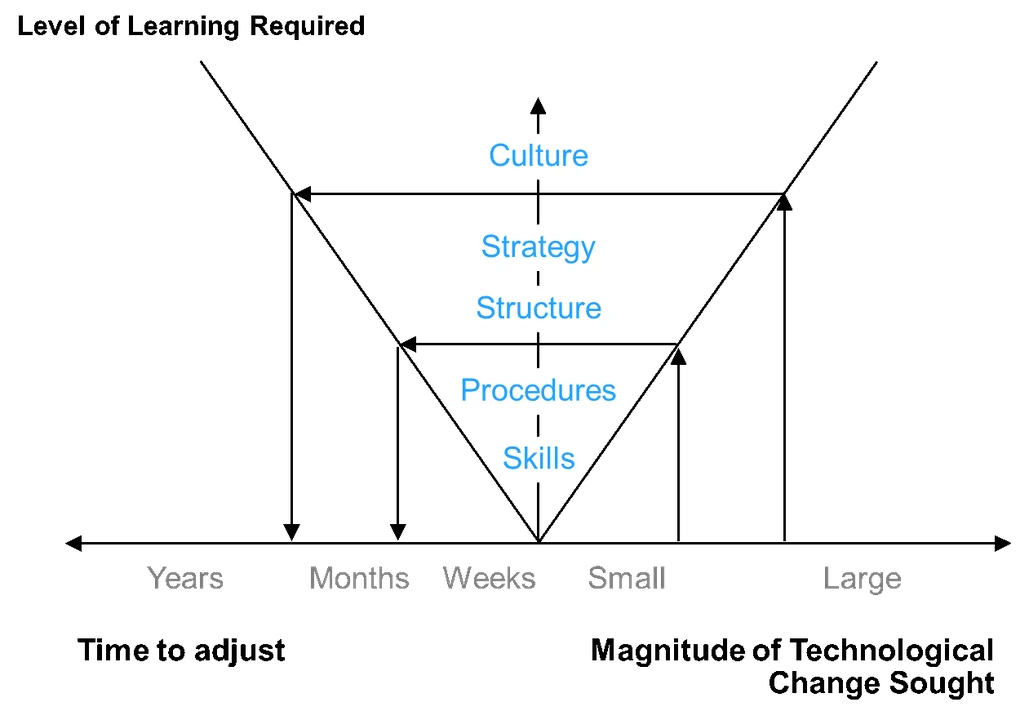
Source: Adapting Your Technological Base: The Organizational Challenge by Paul S. Adler and Aaron Shenhar.
The largest, most difficult technologies to adopt are those that require the organizational culture (assumptions and values) to change. A culture change requires change to strategy, structure, procedures, and skills. Adler's Magnitude of Technology Change model is one that easily communicates to senior management the scope of change and the reason that many technology changes require longer than might be assumed.
Adler's Magnitude of Technology Change model has two implications for digital natives and digital immigrants. The first, more obvious, implication is that digital natives learn how to use new technology in different ways than digital immigrants. Their patterns of learning involve more direct engagement with the technology and less traditional (e.g., live, in the classroom) learning modes, which digital immigrants tend to rely on. In a population comprising both groups, the natives are often impatient with face-to-face, lecture-based training events, whereas the immigrants will get frustrated with online tutorials that seem to skip over the conceptual aspects and get right to skill application.
A more subtle difference between the two groups concerns what each perceives as a cultural shift. For example, in the health care industry, digital natives have easily adopted electronic health records, whereas digital immigrants view them as a significant cultural change. An organization that is led by digital natives, but includes a significant number of digital immigrants, may devote fewer resources and less planning to a new technology rollout than needed for successful adoption by the whole organization.
Who will be adopting the new technology?
Interestingly, when characterizing adoption populations, an individual may be categorized as one kind of adopter for one technology and a completely different kind of adopter for another technology.
The Everett Rogers Adoption Population model has been around for more than 40 years. In the 1990s, Geoffrey Moore in Crossing the Chasm highlighted its utility for managing high-tech marketing, and he also updated it to include the concept of a chasm between two particular categories of adopters.
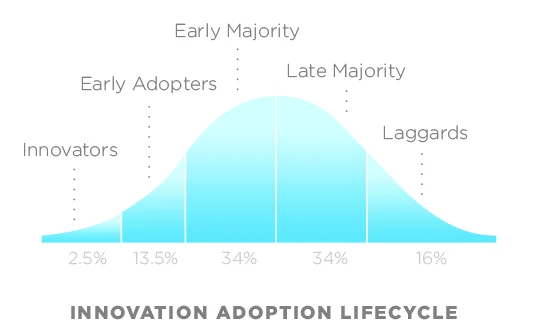
Source: A graph of Everett Rogers Technology Adoption Lifecycle model.
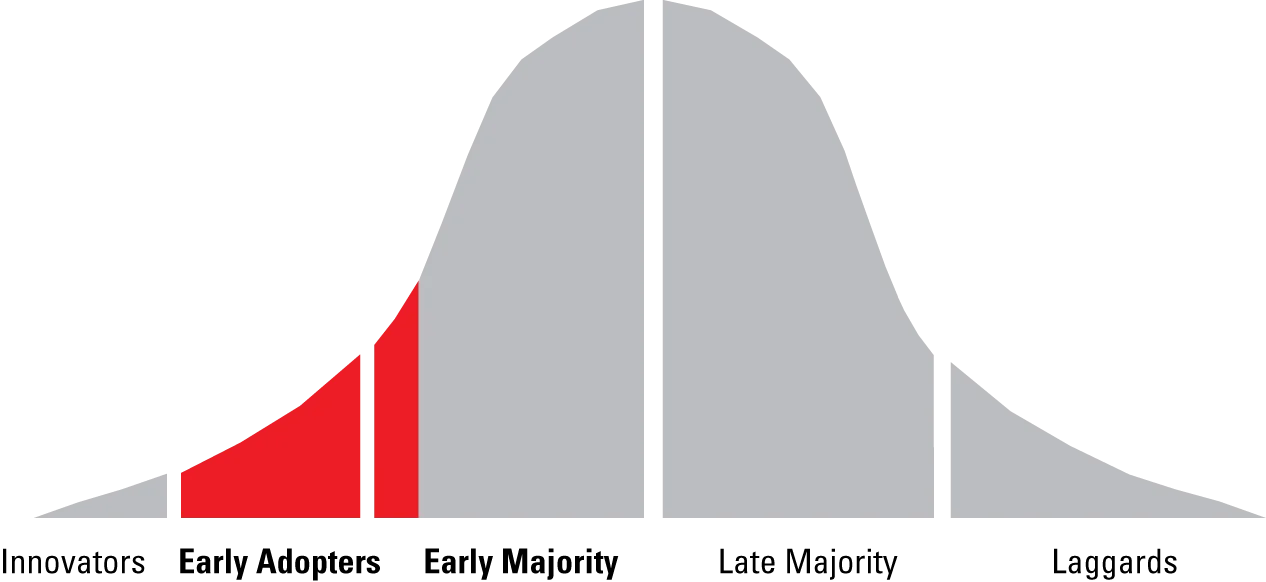
Source: Crossing the Chasm, 3rd edition by Geoffrey Moore.
The model contains five basic categories:
- Innovators require little prodding to try a new technology and generally require little support for making it work. They are often serially enthusiastic about multiple technologies of a particular type, always looking for the next big thing.
- Early adopters look for strategic advantage from new technologies but are not adopting a new technology for its own sake. They are willing to accept some risks associated with adoption, but only if they can see how the technology fits into the larger picture of their market or their mission. They don't require a fully baked solution, but they may need some support (usually consulting). They are often called visionaries.
- The early majority are pragmatic about using new technologies. If a new technology is cooked enough and is visibly useful to support their mission, they will consider adopting it, but they want to be fairly certain of the return on investment they can expect. Even if the technology isn't fully proven in their context, it should be proven in at least one context they consider relevant. They are often called pragmatists.
- The late majority only make a shift to a new technology when it has been proved and is well-supported. They want training, checklists, and tools to be tuned to their context and role. The less they have to change their practices to accommodate the technology, the better. They are often called Main Street.
- Laggards will avoid adopting a new technology, often at great cost to themselves. They will continue to see problems in the technology long after it has been adopted by the mainstream and will sometimes make serious shifts in their job or other situation to avoid a technology they don't want to adopt.
Between the early adopter and early majority populations, Moore added the concept of the "chasm," which represents the significant difference in how to communicate about a technology with early adopters versus how to talk with early majority adopters. Technologies that seem to stall in their adoption journey are often victims of the chasm. If the technologists do not change their communication and marketing strategy for the early majority, their technology will often fail at being adopted across a wide population. Although it may be tempting to classify digital natives as early adopters and digital immigrants as early majority, it's often not that clear-cut. Some digital immigrants are early adopters of certain types of technologies that they can easily envision supporting their organizational or individual goals. In other situations, a digital native may not see the cost/benefit of a new technology if the current technology it replaces meets their organizational or individual needs.
Individuals will not always be in the same category for every technology.
In this model, digital natives are less likely to be laggards in information technology settings, as long as those settings are related to the technology environments they are familiar with already. For example, digital natives typically pick up smartphone-based apps more readily than digital immigrants. As with the larger population, not all digital natives will consistently fall into the category of innovators or early adopters, so it's still worth investigating the population that you're dealing with in any particular instance.
Adoption Commitment Curve Model
Once we know who the adopters are and the size of the adoption, the next model that helps us move forward is Adoption Commitment Curve model introduced by Daryl R. Conner and Robert W. Patterson. This model is based on research on how individuals learn new things, a key element in adopting most technologies.
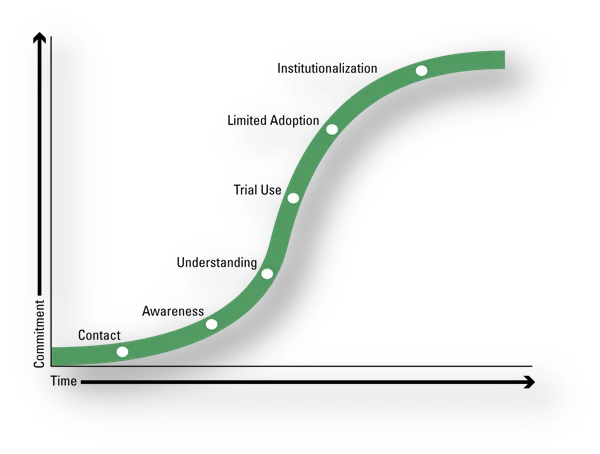
Source: Building Commitment to Organizational Change (18-30) by Daryl R. Conner and Robert W. Patterson.
The stages for the Commitment Curve are:
- Contact. We first learn about the name of the technology and its general purpose. In the contact stage, we probably can't expand the acronym associated with the technology, but we have a vague idea of who is promoting it and why.
- Awareness. We are learning more about the general attributes of the technology and who might be candidates for adopting it. We can tell someone what the acronym means and provide a high-level description of it, but can't yet articulate how the technology would work in our particular environment. (From the viewpoint of designing mechanisms to transition a group from one stage to another, contact and awareness are often treated together, because they use similar mechanisms.)
- Understanding. We not only understand what the technology is but also understand how we could apply it in our own environment. We haven't yet tried it, but, if pressed, we know how we would do it. If we can't get to this stage, we are not likely to get very far with actual adoption.
- Trial use. We try using the technology for our mission or operational goals. The inflection in the curve increases as we move into this stage, because the shift into trial use takes a large amount of energy and support.
- Adoption or limited adoption. This is the stage at which we have proved to ourselves that the technology works (trial use has provided us with technical feasibility pilots), and we are now performing adoption feasibility pilots--rolling out the technology, along with appropriate support, to the intended populations for adoption. The reason this is sometimes called limited adoption is that the technology hasn't made it into the organization's policy or infrastructure yet, and it could be displaced if something better came along or something disastrous occurred when using it.
- Institutionalization. This is the stage when the new technology becomes the new status quo within the organization. The accompanying policy and infrastructure support is in place, making it difficult to back out of the technology. Generally speaking, we would resist abandoning the technology because it now supports our work.
- Internalization. This is the stage (not shown in the graphic) at which we have become so invested in the technology that we would seriously sabotage any attempts to remove it, and might even leave the organization if the technology were removed. In terms of technology adoption, most organizations are not seeking internalization, because they know that most technologies will have to be replaced in the future, and a technology that is internalized is very difficult to displace. The main reason for understanding this stage is to be able to recognize the symptoms that an organization has internalized the technology we are trying to replace.
Generally speaking, communication mechanisms help people move from contact through awareness to understanding. Although communication mechanisms are also used for later stages, the character of support changes to implementation support mechanisms for trial use through adoption and institutionalization.
This model can be very helpful to diagnose anomalies in an adoption setting. Organizations often try to move too quickly to trial use, without helping employees understand what this technology means to them and their work. Conversely, organizations also ignore providing the implementation support their constituents need, focusing instead on the conceptual benefits of the technology. When I am working with an organization on adopting new practices or technologies, I can quickly determine their focus by looking at the kinds of transition mechanisms they have made available to support the transition.
This model highlights significant differences in how digital immigrants and digital natives approach new technology adoption settings. Since the Adoption Commitment Curve is essentially a learning model built on the observations of digital immigrants (the original model was published in 1981, when there were no digital natives yet), it's worth thinking about how it might differ when applied to digital natives.
For example, consider user manuals as a transition mechanism. Digital immigrants are more likely to use the sections beyond "Getting Started." Digital natives are more likely to use trial and error until they understand the concepts that guide how the system is used.
Another example is training approaches. As mentioned before, face-to-face, synchronous learning events may be better appreciated by digital immigrants, but shorter, asynchronous webcasts and videos may appeal more to digital natives.
How do we get people from here to there?
In 1997, Jerry Weinberg published the fourth volume in his Quality Software Management series, subtitled Anticipating Change. In that volume, Weinberg introduced a model of change that has proved to be useful at guiding organizations through a significant technology change process. Generally termed the Satir Change Model (named after its initial author, Virginia Satir), it looks at change from the viewpoint of those trying to change their own behavior.
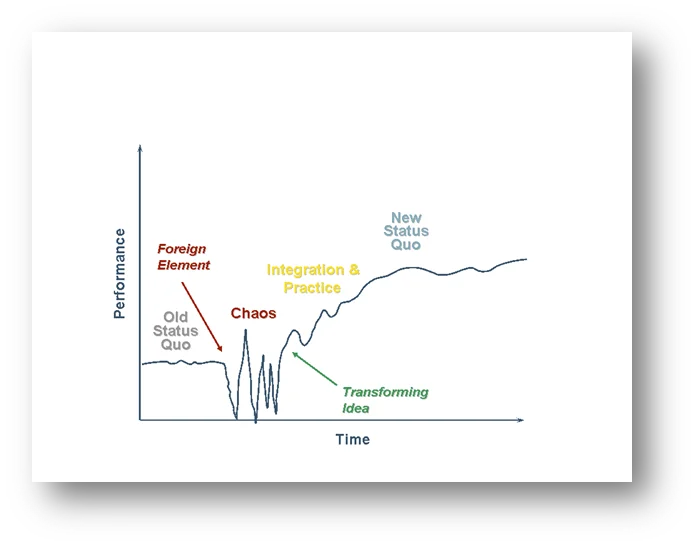
Source: 4th volume in Jerry Weinberg's Quality Software Management series, Anticipating Change.
Prior to introducing a change, whether it is understood or not, there is a status quo of performance that has some baseline variation but is generally stable. When a foreign element is introduced (a technology change, in this case, though it could also be an organizational restructuring or market change), the individual or group goes into a period termed chaos, which is characterized by high variability in performance. During this period, individuals and groups try to determine the character of the change and whether or not they can or should adopt it. They also seek ways to make the change work within their overall framework of performance.
What's called the transforming idea arises when the individual or group figures out how to make the change work for them. We might assume that the transforming idea is the point at which we can realize the improved, more stable performance the foreign element was introduced to provide. We would be wrong: the transforming idea, typically, is something that will require new support mechanisms (see prior section) and new behaviors to ensure the new technologies work (technical feasibility) and that they can be adopted by those whose cooperation is needed for the change to be successful (adoption feasibility). During this stage, performance tends to improve and not vary quite as much as during the chaos stage, but without this the transforming idea, it's unlikely that a new, stable, status quo can be reached that maximizes the impact of the technology change on performance.
A key to moving through chaos, not surprisingly, is authentic communication about the conditions that led to the need for the change--one of the hardest things to achieve in large organizational or technology change.
The Satir Change Model works well in concert with the Adoption Commitment Model. Many of the communication mechanisms needed for individuals and groups to understand the Adoption Commitment Model are the same things that help individuals and groups move through the Satir Change Model's chaos to the transforming idea stages.
In terms of digital immigrants and digital natives, the Satir Change Model is probably the least impactful. In my observation, digital natives tend to move faster through integration and practice with less implementation support than digital immigrants. However, when it comes to practice-based technologies, like adopting new security practices or Agile methods, the main difference lies in the character of support they respond to, rather than how quickly they move from one state to the next.
Wrapping Up and Looking Ahead
Five questions, five models that support understanding a new technology and the implications it has for an organization. The five models detailed in this post can still help us analyze and implement new technologies. But in addition to relying on these classic models, we also need to reflect on new ideas that help us to adopt something new. For example, in recent years there has been significant research on the effects of multi-tasking (the favored term now is "task switching") on employee productivity. Digital natives often have a perception that multi-tasking makes them more productive. This, however, is not borne out by empirical research. To the contrary, research has produced the consistent result that individuals who perceive themselves as good multi-taskers actually are less productive than those who focus on serial task completion. In the next post in this series, I will examine how we take advantage of insights like this when planning technology adoption with a mix of digital native and digital immigrants in the same adoption population.
Additional Resources
The book CMMI Survival Guide: Just Enough Process Improvement provides additional details on using all but one of the models listed here. Don't let the "CMMI" in the title fool you. Even though it was written with CMMI as an example, the second half of the book was meant to provide practical advice on using technology adoption research in practical ways. (I know, I'm one of the co-authors!) Even though it's an older resource, the information is still relevant outside the CMMI adoption context. To learn more about some of these models in relation to adopting Agile practices in the Department of Defense, see the SEI Technical Note, Agile Adoption: Selected DoD Acquisition and Management Concerns.
Get updates on our latest work.
Sign up to have the latest post sent to your inbox weekly.
Subscribe Get our RSS feedGet updates on our latest work.
Each week, our researchers write about the latest in software engineering, cybersecurity and artificial intelligence. Sign up to get the latest post sent to your inbox the day it's published.
Subscribe Get our RSS feed