Collaborative Solution Earns Top-Five Spot in DIUX Challenge
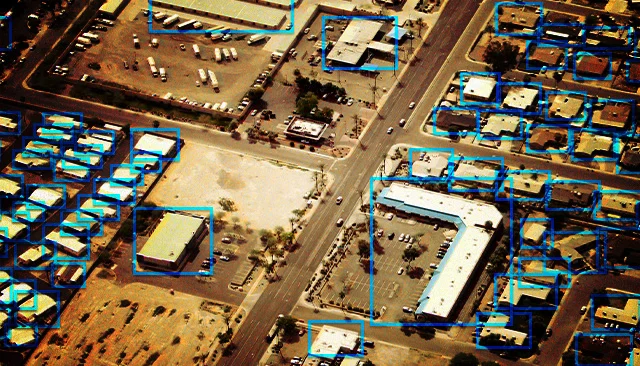
• Article
November 15, 2018—A team of researchers from the Carnegie Mellon University and the University of Pittsburgh scored a top-five finish in the 2018 Defense Innovation Unit Experimental (DIUx) xView Detection Challenge. The challenge, conducted by the Pentagon in partnership with the National Geospatial-Intelligence Agency, sought innovative uses of computer vision techniques in a disaster response scenario to more quickly and accurately read satellite data imagery. The Pittsburgh team applied a “chipping” technique to earn one of the competition’s top scores.
The team included Ritwik Gupta, a machine learning researcher at the Emerging Technologies Center at the Carnegie Mellon University Software Engineering Institute; 2d Lt Alex Fulton, a graduate student at the U.S. Air Force Institute of Technology and Carnegie Mellon’s Information Networking Institute; and Kaylene Stocking, an undergraduate student at the University of Pittsburgh.
According to DIUx organizers, “xView is one of the largest publicly available datasets of satellite imagery.” It contains complex scenes from around the world and focuses on humanitarian assistance and disaster relief tasks. This year, the xView Detection Challenge looked to advance progress in the field of computer vision by identifying solutions that would
• reduce minimum resolution for detection
• improve learning efficiency
• enable discovery of more object classes
• improve detection of fine-grained classes
“Making sense of satellite imagery can be an enormous challenge, especially when the area involved is large, time is of the essence, and the objects populating that area are many and diverse,” said Gupta. “Manual analysis methods are slow and eat up far too many analyst hours.”
Gupta explained that the large image size, minute differences in class labels, and the density of objects contained in the xView dataset images make it difficult to accurately localize and classify objects using existing neural network techniques—the limitations associated with memory and processing-time are too great. However, Gupta and his collaborators were able to overcome some of these limitations by employing a chipping technique that split the images into 300-pixel chips that overlapped by 50 percent.
“Each chip was responsible for detecting a unique area in the image,” said Gupta, “but could use part of other nearby chips to provide better context and improved detection accuracy.”
The team found that this continuous-context scheme not only improved detection performance, but promises to be more feasible for real-world detection in very large satellite images. The effectiveness of the team’s detection technique was validated by the xView Challenge scoring system which automatically scored submissions based on accuracy of classification.
To learn more about the xView Detection Challenge, visit http://xviewdataset.org/.