Join the SEI and White House OSTP to Explore the Future of Software and AI Engineering
PUBLISHED IN
Software Engineering Research and DevelopmentThe Software Engineering Institute (SEI) and the Networking and Information Technology Research and Development Program in the White House Office of Science and Technology Program will co-host an upcoming workshop to inform a community strategy for building and maintaining U.S. leadership in software engineering and artificial intelligence (AI) engineering. The workshop will be held in person at the National Science Foundation in Alexandria, Virginia, on June 20 and 21. Registration for the event closes June 16.
The event will gather thought leaders from federal research funding agencies, research laboratories, and mission agencies, as well as relevant commercial organizations, to explore the fundamental research needed to advance the theory and practice of software engineering, as well as enable the reliable development and trustworthy use of AI in the next generation of software-reliant systems. Speakers will include engineering and research leaders from commercial organizations like Google and Facebook, nine federal agencies, and eight universities.
Using the SEI’s national study on Architecting the Future of Software Engineering: A National Agenda for Software Engineering Research and Development as a starting point, participants will identify and explore important research areas for the future of software engineering that are critical for multidisciplinary research. The workshop focuses on exploring critical needs and priorities at the intersection of software engineering and AI engineering, including (but not limited to) the following topics:
- Will AI change the software engineering lifecycle? Where can AI-enabled capabilities make most difference in improving productivity and quality?
- What would it mean for AI to become a “trusted partner” in software engineering? How will we know when it can be trusted and when it can’t?
- What kinds of software and AI-enabled capabilities will government agencies need in the future? What gaps and risks exist in acquiring them and what are ways to address these issues?
- What are your proposals for the truly transformational Research & Development thrusts that will help engineer the intelligent systems of the future?
The national agenda for software engineering summarized in a previous blog post envisioned some of the advances we are observing today, especially with respect to advances in generative AI. This blog post builds on that initial one demonstrating the links with some of the current developments and describes our vision of the future of software engineering.
The Future of Software Engineering: A Guiding Vision and a Look at Possible Scenarios
Our guiding vision for the future, which is described in the findings of the report and elaborated on below, is one in which the current notion of the software development pipeline is replaced by one where humans and software are trustworthy collaborators that rapidly evolve systems based on programmer intent and continuous assurance. We explore how that might look in some scenarios we’ve imagined for the report (see page 19), and include a few of those here because we think they point toward an exciting future.
For example, consider this scenario:
The days of endless requirements and design reviews are gone. A joint team of aeronautical engineers, pilots, software engineers, and associated AI tools together design the next space-capable craft by pitching ideas, which are turned into viable designs based on access to extensive codified knowledge about cyber-physical systems, as well as the limitations of physics. These designs are displayed in real time, and the team compares defensive and maneuverability capabilities on the fly using real-time simulations of representative missions, multiple alternatives generated at ease using hybrid AI techniques and input from large-language models (LLMs). The final design is selected based on the most desirable balance of cost, capabilities, and timeline. Today’s notion of a software development lifecycle might seem almost archaic compared to this fluid, iterative, and largely automated process.
In the context of this and other emerging and future scenarios, elegant software will no longer refer solely to cleverly designed programs. Instead, it will result from humans working with automated and AI systems to implement the best ideas imaginable in the most timely, affordable, ethical, and secure ways. Who can program and create these complex systems will naturally expand as well. Our conversations with computers will take place in the language of our domains instead of traditional computer languages. For example, computational biologists will develop software capabilities by expressing their ideas and intentions about sequencing and genes to intelligent tools backed by robust LLMs, rather than by learning Python. Specialists of all types will be needed to inform the computer properly, and how they interact will look significantly different than it does today.
The use of simulation and emulation may turn today's entire notion of test and evaluation into an immersive experience. Imagine that a new hardware configuration and software capabilities are planned for a series of space assets. In a fully immersive virtual reality environment, the changes are emulated with the full telemetry of the current assets feeding the environment. Engineers can view the new space configuration from any vantage point and not only in a visual range. All the available data and metadata from the current environment is also presented in real time. Where the desired effect is not what was anticipated, engineers and subject matter experts work collaboratively with intelligent tool chains to make changes and immediately see the impact on the holistic space environment. Moreover, numerous additional stakeholders are observing and manipulating the same environment in a shared experience. Communication between these stakeholders—enabled by many types of media and a shared decision process—ensures that the system as a whole minimizes unintended and/or undesired emergent behaviors. This same environment will be used once changes are made to support operator training and real-time mission rehearsal.
After these systems are deployed, they will also be much more adaptable and integrated. Consider a scenario that involves a special forces team on a deployment, and imagine a firefight breaks out. The squad is caught off guard, communications have been disrupted, and they’re unsure of the weapons being employed against them. Fortunately, they are teaming with a set of micro unmanned aircraft systems that proactively set up a mesh network using alternate communications channels to re-establish contact with headquarters. After that network is established, the squad directs the devices to observe and profile the weapons on the battlefield covertly and provide mitigation options while they take cover. As a result, they are not only able to overcome the novel threat locally but also feed their real-time experience to other units at the tactical edge who could be at risk. To make this scenario a reality, software engineers will need to design architectures that are nimble and leverage intelligent battle management tools that enable adjustments to systems based on data from operational sensors and other input from users in the field. Organizations such as Palantir are already working toward similar scenarios for the use of AI. Their demo video shows how their platform can use AI to display and analyze intel on enemy targets, identify potentially hostile situations, and propose battle plans.
While the exact roles that intelligent algorithms, tools, and humans will have is not yet determined, the importance of software engineering to our vision is clear. As software engineers continually interact with smart software assistants (including but not limited to LLMs), computers and humans will be able to do what they both do best. Working in this way, possibilities that we cannot even imagine today will become reality. The research in this report provides the essential groundwork for advancing the discipline of software engineering to ensure that the necessary framework is in place to maximize the advantages these future opportunities can provide.
To achieve this vision, we anticipate the need for new development and architectural paradigms for engineering future systems. As discussed in Architecting the Future of Software Engineering: A Research and Development Roadmap, the SEI developed six research focus areas in close collaboration with our advisory board and other leaders in the software engineering research community. The following figure shows those areas, along with a list of research topics to undertake, and then short descriptions of each of the research focus areas follow.
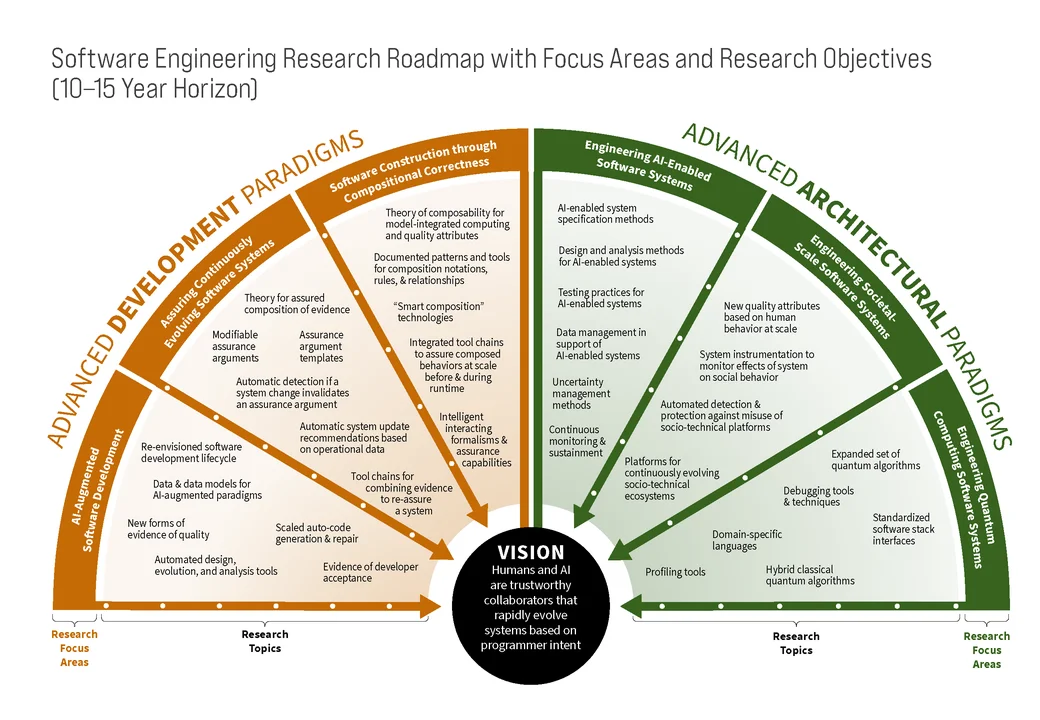
AI-Augmented Software Development. At almost every stage of the software development process, AI holds the promise of assisting humans. AI-based (and other) automated tools are increasingly being used to improve the efficiency of software engineers and reduce their cognitive load. By shifting the attention of humans to the conceptual tasks that computers are not good at and eliminating human error from tasks where computers can help, AI will play an essential role in a new, multi-modal human-computer partnership. To reach this important goal, we need to re-envision the entire software development process with increased AI and automation tool support for developers.
A key challenge will be taking advantage of the data generated throughout the lifecycle. The focus of this research area is on what AI-augmented software development will look like at each stage of the development process and during continuous evolution, where AI will be particularly useful. AI-enabled and other automated capabilities will enable developers to perform their tasks better and with increased quality and accuracy. An important open research question is how these tools can also enable creating different and more effective and robust workflows.
Assuring Continuously Evolving Software Systems. When we consider the software-reliant systems of today, we see that they are not static (or even infrequently updated) engineering artifacts. Instead, they are fluid—meaning that they are expected to undergo almost continuous updates and improvements and be shown to still work. As LLMs are taking software development by storm there are two key changes occurring that influence assurance. This new generation of tools (an increasing number of which are empowered by LLMs) is increasingly adept at generating error-free code, especially for trivial implementation tasks. Moreover, these tools are also increasingly capable of generating surprising recommendations that may themselves create additional assurance concerns. The goal of this research area therefore is to develop a theory and practice of rapid and assured software evolution that enables efficient and bounded re-assurance of continuously evolving systems, recognizing that generated systems may have less trivial errors while including other harder-to-spot concerns that may create more complicated assurance problems.
Software Construction through Compositional Correctness. As the scope and scale of software-reliant systems continues to grow and change continuously, the complexity of these systems makes it unrealistic for any one person or group to understand the entire system (or system-of-systems). It is therefore necessary to integrate (and continually re-integrate) software-reliant systems using technologies and platforms that support the composition of modular components. This composition is particularly hard since many components reused from existing elements were not designed for integration or co-evolution. The goal of this research area is to create methods and tools that enable the intelligent specification and enforcement of composition rules that allow (1) the creation of required behaviors (both functionality and quality attributes) and (2) the assurance of these behaviors at scale.
Engineering AI-enabled Software Systems. AI-enabled systems, which are software-reliant systems that include AI and non-AI components, have some inherently different characteristics than those without AI, particularly with respect to non-determinism and emergent behavior. These include but are not limited to emergent behavior, extreme dependence on data, and designing for explainability and fairness. Above all, however, AI-enabled systems share many parallels with developing and sustaining conventional software-reliant systems. Many future systems will likely either contain AI-related components, including but not limited to LLMs, or will interface with other systems that execute capabilities using AI. Many existing and legacy systems will also face pressures to evolve and include AI-related components. Continuous monitoring and rapid change management will be key in the development and sustainment of such systems. This research area, therefore, must focus on exploring which existing software engineering practices can reliably support the development of AI systems and the ability to assess their output, as well as identifying and augmenting software engineering techniques for specifying, architecting, designing, analyzing, deploying, and sustaining AI-enabled software systems.
Engineering Socio-Technical Systems. Societal-scale software systems, such as today’s ubiquitous social media systems, are designed to engage and influence users. A key challenge in engineering societal-scale systems is predicting outcomes of socially-inspired quality attributes that arise when humans represent integral components of the system. As generative AI makes rapid progress, these societal-scale software systems are also prone to abuse and misuse by AI-enabled bad actors via techniques such as chatbots imitating humans, deep fakes, and vhishing. The goal is to leverage insights from other fields, such as the social sciences, as well as regulators and legal professionals to build and evolve societal-scale software systems that consider these challenges and attributes.
Engineering Quantum Computing Software Systems. Advances in software engineering for quantum are as important as the hardware advances. Systems are increasingly heterogeneous computing environments and quantum computing is one example of new and emerging computational models. The goals of this research area are to first enable the programming of current quantum computers more easily and reliably and then enable increasing abstraction as larger, fully fault-tolerant quantum computing systems become available. A key challenge is to create approaches that integrate different types of computational devices into predictable systems and a unified software development lifecycle.
Research and Enactment Recommendations to Catalyze Change
Catalyzing change that advances software engineering will yield more trustworthy and capable software-reliant systems. The research focus areas shown in Figure 1 led to a set of research recommendations needed to catalyze change, which are followed by enactment recommendations that focus on people, investment, and sustainment are needed.
The following research recommendations address challenges, such as the increasing use of AI, assuring changing systems, composing and re-composing systems, and engineering socio-technical and heterogenous systems:
- Enable AI as a reliable system capability enhancer. The software engineering and AI communities should join forces to develop a discipline of AI engineering to ensure reliable development of AI models and incorporating those to trustworthy systems. This collaboration should enable the development and evolution of AI-enabled software systems that behave as intended and enable the use of AI as an effective and trusted software engineering workforce multiplier.
- Define practices for AI-Augmented Software Development: The software engineering research community should focus on emerging and envisioned automated tools, including but not limited to those powered by generative and other AI capabilities, in two dimensions. One dimension, which we call do better, will be to improve existing development tasks without changing their workflow but improving their scale and correctness. Another important dimension will be to investigate how tasks can be done differently envisioning new software development task workflows. Special attention should also be paid to prompt engineering, with the development of specific technical skill sets that enable humans to effectively develop and refine prompts that program LLMs.
- Develop a theory and practice for software evolution and re-assurance at scale. The software engineering research community should develop a theory and associated practices for re-assuring continuously evolving software systems. A focal point for this research is an assurance argument, which should be a software engineering artifact equal in importance to a system’s architecture, that ensures small system changes only require incremental re-assurance. Understanding ways in which emerging AI-enabled tools can (and cannot) facilitate these assurance arguments will help inform this research.
- Develop formal semantics for composition technology. The computer science community should focus on the newest generation of composition technology to ensure that technologies, such as model-driven development and dependency-injection frameworks, preserve semantics through the various levels of abstraction that specify system behavior. These semantic preservations will allow us to reap the benefits of development and integration at scale via intelligent composition while achieving predictable runtime behavior.
- Mature the engineering of societal-scale socio-technical systems. The software engineering community should collaborate with social science communities to develop engineering principles for socio-technical systems. Theories and techniques from disciplines such as sociology and psychology should be used to discover new design principles for socio-technical systems, which in turn should result in more predictable behavior from societal-scale systems.
- Catalyze increased attention on engineering for new computational models, with a focus on quantum-enabled software systems. The software engineering community should collaborate with the quantum computing community to anticipate new architectural paradigms for quantum-enabled computing systems. The focus should be on understanding how the quantum computational model affects all layers of the software stack.
The recommendations above focus on scientific and engineering barriers to achieving change. The following enactment recommendations focus on institutional obstacles, including economic, human, and policy barriers.
- Ensure investment priority reflects the importance of software engineering as a critical national capability. The strategic role of software engineering in national security and global market competitiveness should be reflected in national research activities, including our upcoming workshop. These research activities should recognize software engineering research as an investment priority on par with chip manufacturing and AI with benefits to national competitiveness and security. Software engineering grand challenges sponsored by the Defense Advanced Research Projects Agency (DARPA), the National Science Foundation, and FFRDCs are also suggested.
- Institutionalize ongoing advancement of software engineering research. Sustained advancements in software engineering require institutionalizing an ongoing review and reinvestment cycle for software engineering research and its impact on software engineering practice. Maintaining national software engineering proficiency requires entities that fund research and institutes working with industry and government leaders in the software engineering community to periodically review the state of software engineering.
- Develop a strategy for ensuring an effective workforce for the future of software engineering. Currently, software engineering is performed by a broad collection of people with an interdisciplinary skill set that does not always include formal training in software engineering. Moreover, the nature of software engineering is changing in response to the fluid nature of software-reliant systems. We need to better understand the workforce that is required and what we must do to foster its growth. The software engineering community, software industry, and academic community should create a strategy for ensuring an effective future software engineering workforce. Public excitement in LLMs is driven by the promise that they may enable a workforce with less formal training in software engineering methods and tools to make adequate progress on complex software engineering tasks. Making this a reality will require significant research in establishing guard rails into these models to avoid unintended risks, educating the new (and existing) workforce in using effective interaction techniques through prompt engineering, and ensuring that experts are always in the loop guiding the process from the creation of the models to their application.
Architecting Future Systems Requires Software Engineering Advances
Software continues to grow, without bounds, in capability, complexity, and interconnection. To make future software systems safe, predictable, and evolvable, the software engineering community—with sufficient investment from private and public sources—must work together with other key stakeholders to advance the theory and practice of software engineering, as well as enabling the reliable development and trustworthy use of AI in the next generation of software-reliant systems.
As the Honorable Heidi Shyu, Under Secretary of Defense for Research and Engineering wrote in our report:
In the future, we will need rapid composition of new capabilities that can operate in a highly contested and denied environment. Integrating heterogeneous systems seamlessly and rapidly will enable us to stay ahead of threats. We will need to exploit the promise of artificial intelligence to increase capability not only in our fielded systems but also in our development systems. This research roadmap should serve as the starting point for a sustained effort to improve software engineering. The DoD will continue to look to the Carnegie Mellon University Software Engineering Institute as a leader in improving the state of the art and practice in software engineering.
Additional Resources
Download the study Architecting the Future of Software Engineering: A National Agenda for Software Engineering Research & Development.
More By The Authors
More In Software Engineering Research and Development
The Latest Work from the SEI: Counter AI, Coordinated Vulnerability Disclosure, and Artificial Intelligence Engineering
• By Bill Scherlis
PUBLISHED IN
Software Engineering Research and DevelopmentGet updates on our latest work.
Sign up to have the latest post sent to your inbox weekly.
Subscribe Get our RSS feedMore In Software Engineering Research and Development
The Latest Work from the SEI: Counter AI, Coordinated Vulnerability Disclosure, and Artificial Intelligence Engineering
• By Bill Scherlis
Get updates on our latest work.
Each week, our researchers write about the latest in software engineering, cybersecurity and artificial intelligence. Sign up to get the latest post sent to your inbox the day it's published.
Subscribe Get our RSS feed